On Objectivity – and the Bridge to Truth
Statistics are held in high regard. Although the saying goes like “do not trust any statistics you did not fake yourself”, they are often regarded as a prime example of objectivity based on the foundation of large datasets. This view is taken to the extreme when it comes to machine learning: machine learning models are statistical learners. A recently published research article criticizes this view: “the mythology surrounding ML presents it—and justifies its usage in said contexts over the status quo of human decision-making—as paradigmatically objective in the sense of being free from the influence of human values” (Andrews et al. 2024).
The fact that machine learning is seen as an extreme case of objectivity has its origins in the 19th century. Back then, the foundations of our current understanding of objectivity were laid. Human (and fallible) subjectivity was contrasted with mechanical objectivity. At that time, machines were considered to be free from willful intervention, which was seen as the most dangerous aspect of subjectivity (Daston / Galison 2007). To this day, machines – be they cameras, sensors or electronic devices, or even the data they produce – have become emblematic for the elimination of human agency and the embodiment of objectivity without subjectivity. These perceptions persist, and it becomes necessary to explain why common sense continues to attribute objectivity and impartiality to data, statistics and machine learning.
The debate of the 19th century has its revenant today in the discussion about biases. The fact that every dataset contains statistical distortions is obviously not compatible with the attribution of objectivity, which is supposed to be inherent in particular in large datasets. From a statistical point of view, what happens is that large sample sizes boost significance; the effect size becomes more important. On the other hand, “large” does not mean “all”; rather, one must be aware of the universe covered by the data. Statistical inference, i.e. conclusions drawn from data about the population as a whole, cannot be easily applied because the datasets are not established to ensure representativeness (Kitchin 2019). A recent article states with regard to biases: “Data bias has been defined as ‘a systematic distortion in the data’ that can be measured by ‘contrasting a working data sample with reference samples drawn from different sources or contexts.’ This definition encodes an important premise: that there is an absolute truth value in data and that bias is just a ‘distortion’ from that value. This key premise broadly motivates approaches to ‘debias’ data and ML systems” (Miceli et al. 2022). What sounds like objectivity and ‘absolute truth value’ because it is based on large datasets, statistics and machine learning models is not necessarily correct, because if the model is a poor representation of reality, the conclusions drawn from the results may be wrong. This is also the reason why Cathy O’Neil in 2016 described an algorithm as “an opinion formalized in code” – it does not simply offer objectivity, but works towards the purposes and goals for which it was written.
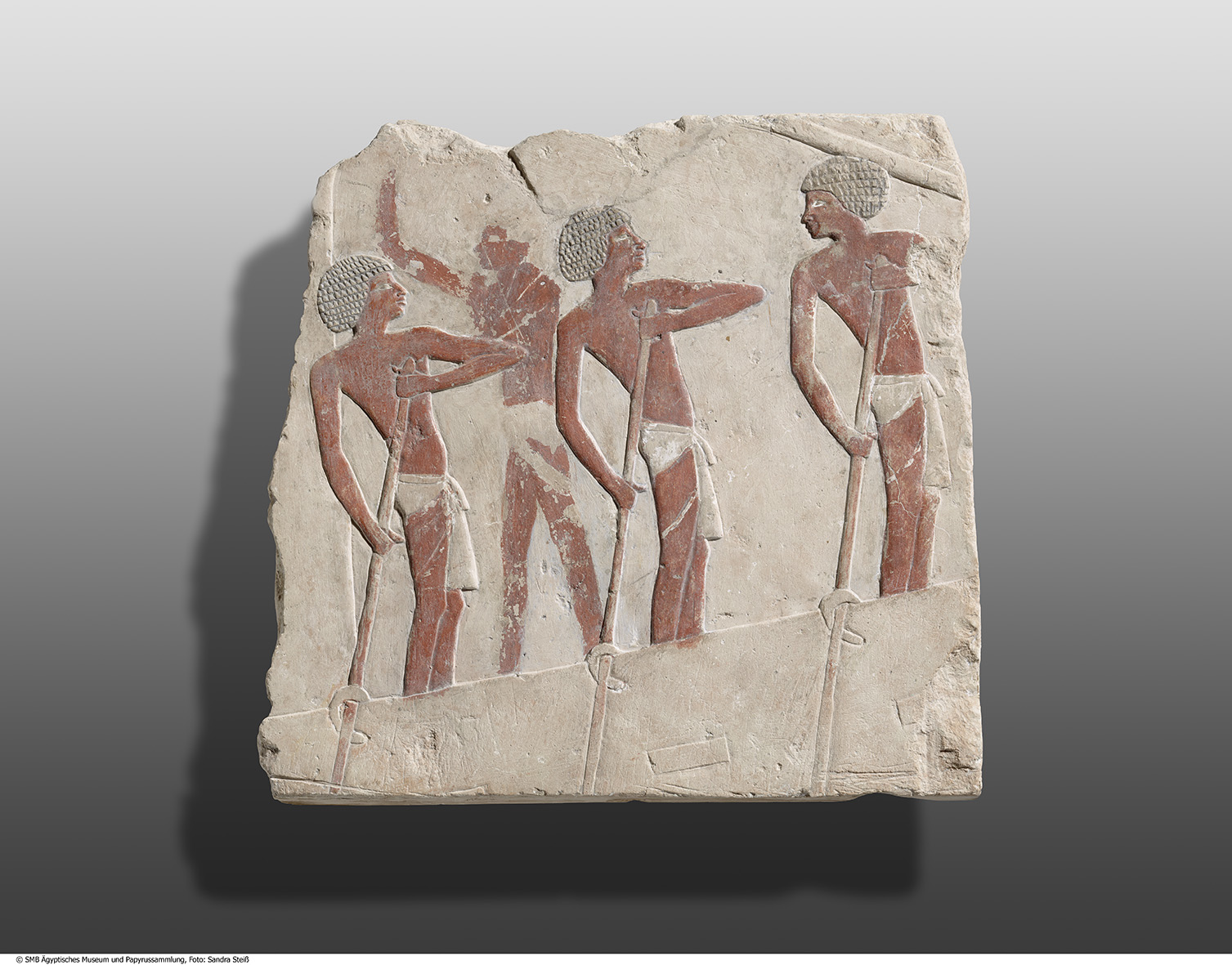
Relief fragment with depiction of rowers, Hatschepsut (Queen, Ancient Egypt, 18. Dynasty). Staatliche Museen zu Berlin, Egyptian Museum und Papyrus Collection. Public Domain Mark 1.0
A historical visualisation of scientists communicating with each other and harmonising their views in the sense of the community being above the individuum?
The fact that scientists – and the machine learning community in particular – still adhere to the concept of objectivity and the objective nature of scientific knowledge is due to the fact that the latter is socially constructed because it is partly derived from collective beliefs held by scientific communities (Fleck 1935/1980). Beyond the activity of the individual researcher, the embedding of research results within a broader scientific discourse shows that scientific research is a collective activity. Much of what is termed ‘science’ is based on social practices and procedures of adjudication. As the historian of science Naomi Oreskes noted in 2019, the heterogeneity of the scientific community paradoxically supports the strength of the achieved consensus: “Objectivity is likely to be maximized when […] the community is sufficiently diverse that a broad range of views can be developed, heard, and appropriately considered.” This was obviously also clear to Miceli et al. when they took position in the debate on biases: “data never represents an absolute truth. Data, just like truth, is the product of subjective and asymmetrical social relations.” Ultimately, the processes that take place within such scientific communities lead to what is referred to as scientific truth. Data, statistics, machine learning and objectivity are embedded in social discourses, and in the last instance, the latter form the bridge to truth.