Openness, Efficiency and Closed Infrastructures
The concept of data spaces, that the European Commission is pursuing, is not only a technical one; it also implies a political constitution. Data spaces such as GAIA-X do not require centralised management. The operation of such a data space can take place within a federation that establishes the means to control data integrity and data trustworthiness. The federation that operates the data space is therefore more like the European Union (i.e. a federation of states) than like a centralised democracy. And trust is not only something that characterises cultural heritage institutions in terms of data and machine learning models. Such institutions fulfil their mission on the basis of the trust that people place in them, a trust that has grown over decades or centuries and is an expression of people’s conviction that these renowned and time-honoured institutions make the right decisions and, for example, make the right choices when acquiring their objects.
The political concept of data spaces thus stands in clear contrast to the hierarchical and opaque structures of big tech companies. With regard to data and machine learning models, a clear centralisation movement can be observed in the relevant corporations (Alphabet, Meta, Amazon, Microsoft) since the 2010s, particularly with regard to research and development and the provision of infrastructure. A study published in 2022 on the values that are central to machine learning research emphasises two insights: firstly, the presence of large tech companies in the 100 most-cited studies published in the two most influential machine learning conferences is massively increasing. “For example, in 2008/09, 24% of these top cited papers had corporate affiliated authors, and in 2018/19 this statistic more than doubled, to 55%. Moreover, of these corporations connected to influential papers, the presence of “big-tech” firms, such as Google and Microsoft, more than tripled from 21% to 66%.” This means that tech companies are almost as frequently involved in the most important research as the most important universities. Putting the consequences of this privatisation of research for the distribution of knowledge production in Western societies into perspective would be worthy of its own studies. On the other hand, the study by Birhane et al. emphasises a value that is repeatedly highlighted in the 100 examined research articles: Efficiency. The praise of efficiency is in this case not neutral, as it favours those institutions that are able to process constantly growing amounts of data and procure and deploy the necessary resources. In other words, emphasising a technical-sounding value such as efficiency “facilitates and encourages the most powerful actors to scale up their computation to ever higher orders of magnitude, making their models even less accessible to those without resources to use them and decreasing the ability to compete with them.”
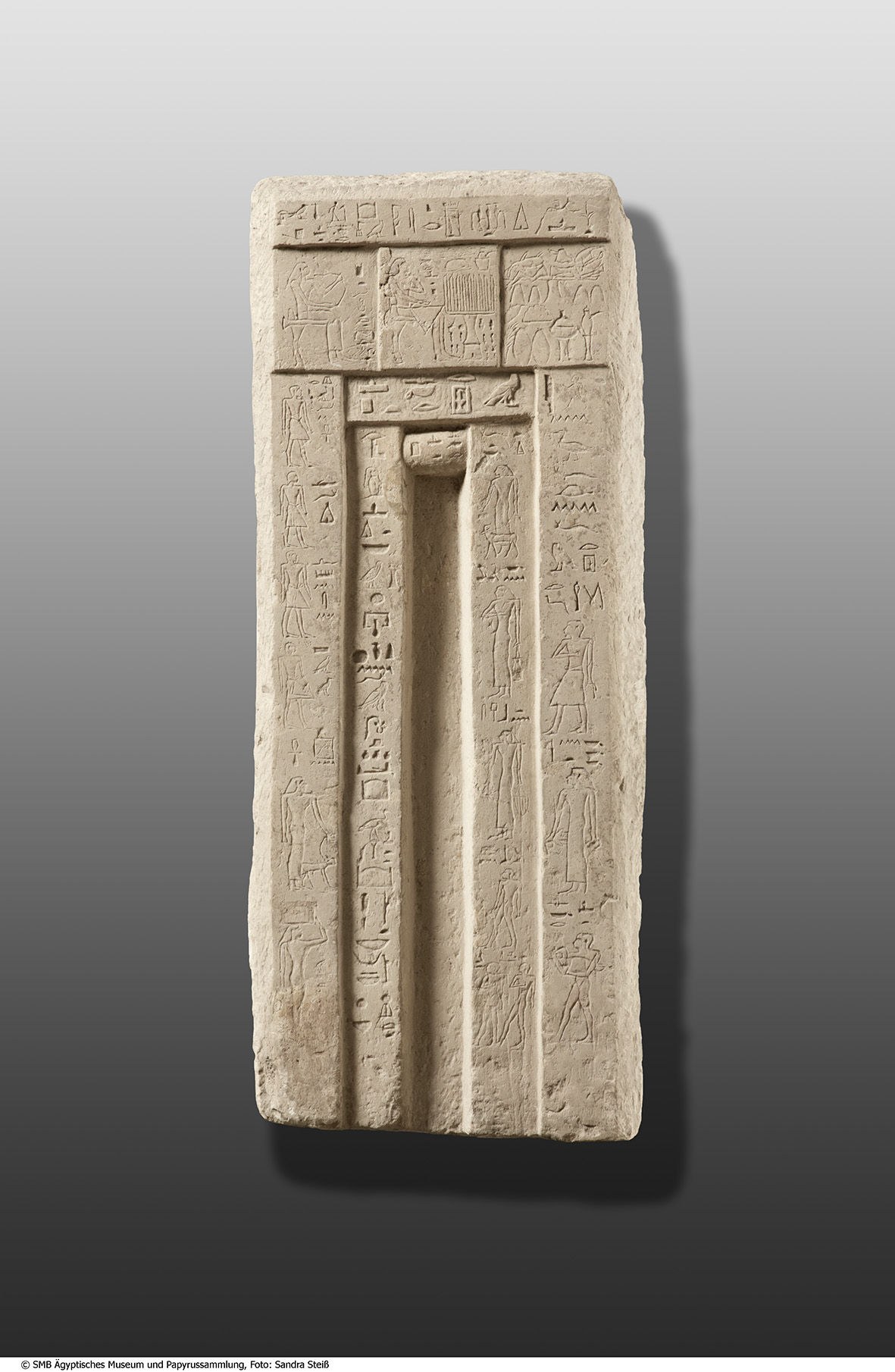
Feigned door of Sokarhotep, Old Kingdom, 5. Dynasty. Ägyptisches Museum und Papyrussammlung. CC BY-SA 4.0.
The feigned door of Sokarhotep symbolises the feigned openness of AI applications provided by big tech
This already addresses the second aspect, the power of disposal over infrastructure. There is no doubt that there is already a “compute divide” between the big tech companies and e.g. elite universities. Research and development in the field of machine learning is currently highly dependent on the infrastructure provided by a small number of actors. This situation also has an impact on the open provision of models. When openness becomes a question of access to resources, scale becomes a problem for openness: Truly open AI systems are not possible if the resources needed to build them from scratch and deploy them on a large scale remain closed because they are only available to those who have these significant resources at their disposal. And these are almost always corporations. A recently published study on the concentration of power and the political economy of open AI therefore concludes that open source and centralisation are mutually exclusive: “only a few large tech corporations can create and deploy large AI systems at scale, from start to finish – a far cry from the decentralized and modifiable infrastructure that once animated the dream of the free/open source software movement”. A company name like “OpenAI” thus becomes an oxymoron.
Against this backdrop, it becomes clear that the European concept of data spaces represents a counter-movement to the monopolistic structures of tech companies. The openness, data sovereignty and trustworthiness that these data spaces represent will not open up the possibility of building infrastructures that can compete with those of the big tech companies. However, they will make it possible to develop specific models with clearly defined tasks that work more efficiently than the general-purpose applications developed by the tech companies. In this way, the value of efficiency, which is central to the field of machine learning, could be recoded.
Leave a Reply
Want to join the discussion?Feel free to contribute!